Table of Contents
Introduction
Machine learning (ML) has become a cornerstone of modern technology, powering everything from personalized recommendations on Netflix to self-driving cars. But what exactly is machine learning and how does it work? We will break down the fundamentals of machine learning. It’s types, applications and challenges, providing a clear understanding of this transformative field.
What Is Machine Learning?
Machine learning is a part of AI that enables system to learn and improve from capable without being explicitly programmed. Instead of relying on static instructions ML algorithms use data to identify patterns make decisions and refine their performance over time. Think of it as teaching a computer to recognize trends in data like how a child learns to identify animals by seeing multiple examples.
What is the importance of machine learning in achieving financial lending sustainability?
The financial industry eagerly looking to adopt AI solutions. It’s important to understand how AI in your business can play an important role in helping your lending operations. Going back many bankers built trust with FICO credit rating systems.
However in addition to continuous innovation and development. the practice of loan interest rates and mortgage loans is constantly changing. FICO which has worked very well in the past is no longer scalable for future practices raising questions among bankers around the world what’s next?
The banking and lending industry is developing at a rapid pace due to new technological innovations, changing consumer indicators, geopolitical dynamics and unpredictable demographic trends.
It was necessary to develop and practice a new form of credit control, banking, credit and mortgage loans. And banks and credit institutions have become interested in AI and ML applications. Machine learning and artificial intelligence in lending and banking have brought new perspectives to fill the limitations of FICO as a system.
Personalized Products and Services
Gone are the days when financial services only meant saving in the bank or taking out a loan. Machine learning expands the scope of financial services to so called consumer financial services. Consumer financial services put consumers and their unique needs at the centre of highly optimized offers. Machine learning enables you to provide consumers with a personal financial advisor who automatically enables them to choose the right spending, saving and investing style based on their personal habits and goals. In finance, machine learning enables the creation of intelligent products that can learn from your financial data and determine what works for you and what doesn’t help you better track your financial activities.
Reduced Transaction Costs
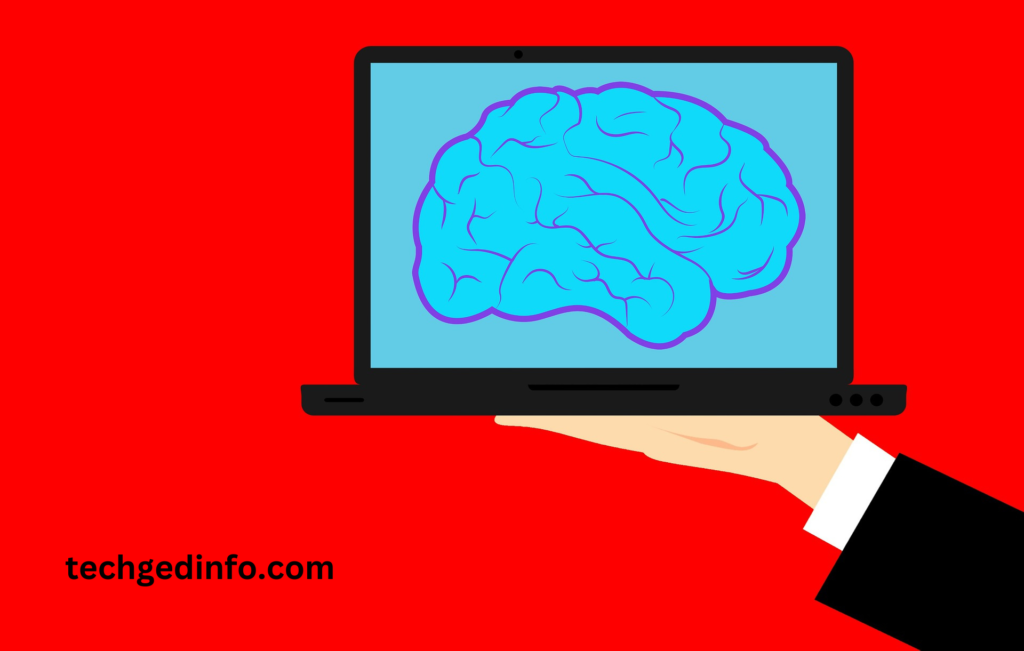
We have all had to experience that, and that’s why we agree with him. Machine Learning in finance has automated processes and drastically reduced customer service costs. Although machine learning has reduced the cost of financial services on the one hand, it has also made the use of financing extremely convenient. Through various digital service channels machine learning is proving effective in attracting large segments of the population to financial services that previously found them cumbersome expensive and time-consuming.
New Management Methods
Machine learning in finance opens up new ways of negotiating for banking and insurance companies. Financial experts are not limited to human opinions that make predictions or recommendations on financial matters. With machine learning in finance, these leaders can ask questions about the machines about their business and these machines can analyse the data and help them to create data. As for the customers, they can manage their accounts without management fees and with high quality as opposed to using the services of a broker. Conventional advice can pay you around 1%.
Fraudulent Pre-releases
Using Machine Learning, you can simulate fifteen situations where fraud or cybercrime can occur. Machine learning in finance is therefore the right way to ensure a safe and fraud-proof financial service environment. Unlike in the past, financial service system designers no longer have to wait to detect fraud and secure the system. Machine learning is helping the financial industry to innovate freely by securing it’s products and services with a continuous understanding of human psychology. Additionally, machine learning in finance also helps in maintaining constant monitoring. Machine learning ensures that all policies, regulations and security measures are strictly followed in the design and delivery of any financial service.
Business Automation
Important decisions in areas such as finance cannot be compromised by the inherent fallibility of human decision making. Machine learning in finance involves detailed analysis, understanding and learning over long periods of time and large amounts of data. Machine learning introduces automation in areas that require high optimization and maintains user security.
Key Concepts in Machine Learning
1. Data: The foundation of Machine Learning is data. Algorithms require vast amounts of structured (e.g., spreadsheets) or unstructured (e.g., images, text) data to learn.
2. Algorithms: These are mathematical models or rules that process data to find patterns. usual algorithms include decision trees, neural networks and regression models.
3. Training: During training, an algorithm analyse labeled data (input-output pairs) to adjust its parameters and improve accuracy.
4. Prediction: Once trained, the model applies its learned patterns to new, unseen data to make predictions or classifications.
Types of Machine Learning
Machine learning can be broadly categorized into three types
Supervised Machine Learning
The algorithm studies labeled data, where each input corresponds to an optimal output. For example, a spam filter trained on emails marked “spam” or “not spam.” Common tasks include regression (predicting numerical values) and classification (categorizing data).
Unsupervised Machine Learning
The algorithm analyse unlabeled data to find hidden structures. Clustering (grouping similar data points) and dimensionality reduction (simplifying data) are key tasks. An example is customer segmentation in marketing.
Reinforcement Machine Learning
The algorithm learns by interacting with an environment and receiving rewards or penalties for actions. This is used in robotics, gaming (e.g., AlphaGo) and autonomous systems.
Applications of Machine Learning
Machine learning drives innovation across industries:
Healthcare: Predicting disease outbreaks or assisting in diagnostics (e.g., detecting tumors in X-rays).
Finance: Fraud detection, digital transactions and loan recovery.
Retail: Personalized recommendations (e.g., Amazon’s product suggestions).
Transportation: Self-driving cars and route optimization.
Entertainment: Content recommendations on platforms like Spotify or YouTube.
Challenges and Limitations on Machine Learning
Despite it’s potential, Machine Learning faces hurdles:
Data Quality: Biased or incomplete data leads to inaccurate models.
Overfitting: The model is now good enough to give you training, but not good enough.
Interpretability: Complex models like deep neural networks are often “black boxes,” making it hard to explain decisions.
Ethical Concerns: Issues like privacy violations and algorithmic bias require careful regulation.
The Future of Machine Learning
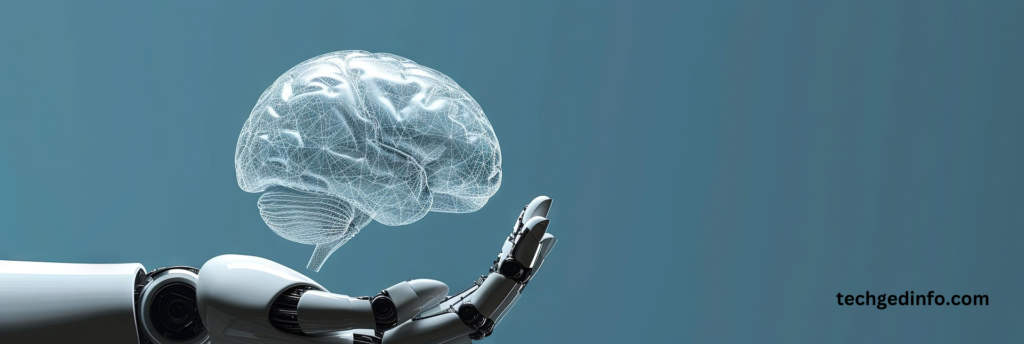
Advancements in computing power, data availability and algorithm design are pushing ML into new frontiers. Emerging trends include:
AutoML: Tools that automate model selection and tuning for non-experts.
Federated Learning: Training models across decentralized devices while preserving privacy.
Ethical AI: Efforts to create transparent, fair and accountable systems.
Conclusion
Machine learning is reshaping how we interact with technology, offering solutions to complex problems across domains. By understanding its principles, types and challenges.
W
e can harness its power responsibly and innovate for a better future. Whether you’re a business leader, developer or curious learner, staying informed about ML is key to navigating the digital age.
1 thought on “What is Machine Learning”